Day 2 :
Keynote Forum
Morgan C Wang
University of Central Florida, USA
Keynote: Improve business operation efficient through the use of automatic intelligent model building system
Time : 09:00-09:35
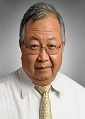
Biography:
Morgan C Wang received his PhD from Iowa State University in 1991. He is the funding Director of Data Mining Program and Professor of Statistics at the University of Central Florida. He has published one book (Integrating Results through Meta-Analytic Review Using SAS Software, SAS Institute, 999), and over 80 papers in refereed journals and conference proceedings on topics including interval analysis, meta-analysis, computer security, business analytics, healthcare analytics and data mining. He is the elected member of International Statistical Association and member of American Statistical Association and International Chinese Statistical Association.
Abstract:
Prescriptive analytics can be used to improve business operation, however, many constraints factors including (i) the shortage of high-quality data analysts; (ii) the time to develop a useful prescriptive model takes very long time; (iii) the lifespan of the prescriptive model is relative short prevent the usage of prescriptive analytics. Automatic intelligent model building system which is capable (a) of building prescriptive model automatically with relatively short time (hours instead of weeks or months); (b) being used effectively by IT personnel with adequate knowledge of data sources; and (c) deploying easily can be used to overcome all the constraints. Thus, it overcomes all shortages of traditional modeling approach and it can be used to improve business operation. A portal type of automatic intelligent model building system has been developed. It is capable of fixing data problems such as missing values, skewness, and high cardinality. It supports neural network, decision trees, gradient boosting, rand forest and many regression algorithms. This system also attempts to open the black box to allow the user to see some insight of the modeling results such as interaction among predictors, important predictors, how to alter predictors to change the predicted values. Two case studies will be discussed to demo the capability of how to use this system to enhance business operation. The first case study is to a precision marketing system. The second case study is on employ management system. The results from both cases studies are very positive and encouraging.
Keynote Forum
Ching Y Suen
Concordia University, Canada
Keynote: Computational Graphology Systems that reveal people's character from their handwritten data
Time : 09:35-10:05
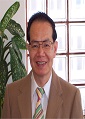
Biography:
Ching Y Suen, Hon. Chair in Artificial Intelligence and Pattern Recognition, Director of CENPARMI (Centre for Pattern Recognition and Machine Intelligence).Concordia University, Montreal, Canada Section Editor and Emeritus Editor-in-Chief of Pattern Recognition, Elsevier Editor of Book Series on Language Processing, Pattern Recognition, and Intelligent Systems, World Scientific Publishing Co. General Chair, Int. Conf. on Pattern Recognition and Artificial Intelligence, Fellow of the Royal Society of Canada, Fellow IEEE, Fellow IAPR Author of 14 books and more than 500 technical papers.
Abstract:
Graphology is a scientific study and analysis of handwriting. It is a practical way of interpreting behavior from examining the peculiarities in handwriting, such as determining people's psychological, social, occupational and medical attributes, as well as their moral stature. Handwriting Analysis has been shown as an effective and reliable indicator of personality and behavior and has become a useful tool for many organizational processes, e.g. recruitment, interviewing and selection, team-building, counseling, and career-planning. This talk will show how handwriting analysis is computerized, what features to look for, methods of investigating the formation of some characters, connectivity between letters, spacing and slant, pen pressure, letter size and placement of strokes, and the presentation and structure of the handwritten document. We shall touch on both computational and psychological aspects in the processing of large volumes of data. The handwriting of famous people and of diversified groups of professionals will be presented, across different languages and over long periods of time. Also, life demos will be given.
Keynote Forum
Shikharesh Majumdar
Carleton University, Canada
Keynote: High-performance data analytics: Platforms, resource management, and middleware
Time : 10:05-10:35
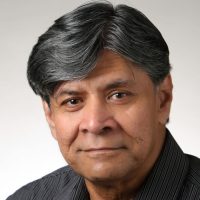
Biography:
Shikharesh Majumdar is a Full Professor and Director of the Real-Time and Distributed Systems Research Centre at the Department of Systems and Computer Engineering in Carleton University, Ottawa, Canada. He is a member of the board for Carleton University Institute for Data Science and of the faculty team associated with Carleton University’s Canada-India Centre for Excellence. He holds a PhD (Computational Science) from University of Saskatchewan, Saskatoon, Canada. His research interests are in the areas of cloud computing, smart systems, high-performance data analytics platforms, operating systems and performance evaluation. He actively collaborates with the industrial sector and has performed his sabbatical research at Nortel and Cistech. He has been the area editor for the Simulation Modelling Practice and Theory journal published by Elsevier (2009-2017). He is a member of ACM, a senior member of IEEE and was a Distinguished Visitor for the IEEE Computer Society (1998-2001).
Abstract:
Enterprises, social networks and smart systems that leverage the Internet of Things technology often lead to large datasets. Data analytics concerns the extraction of knowledge from such raw data. The challenges underlying the processing of such data sets are captured in the 3V characteristics of BigData: Volume, Velocity, and Variety. The first refers to the large size of stored data sets, the second to data in motion streaming from social networks or sensor-based smart systems for example while the third concerns the large variety in data types and formats. High-performance computing platforms such as clusters and clouds are often deployed to address these challenges. Enabling technology that includes parallel processing frameworks and platforms, as well as algorithms for the management of resources in the cloud/cluster, is crucial for performing data analytics in a timely manner. Focusing on such enabling technology this talk will address the various challenges and potential solutions in the context of cloud-based systems for supporting Big Data analytics and smart systems. Issues to be discussed include (a) Management of resources in the context of latency-sensitive data analytics applications such as deadline driven MapReduce jobs and mobile object tracking (video analytics) algorithms. (b) Scheduling techniques for supporting streaming data analytics. (c) Edge-computing based platforms for performing complex event processing in the context of sensor-based streaming applications such as remote patient monitoring. (d) A cloud-based middleware for the unification of geographically dispersed resources required in the management of smart systems such as sensor-based bridges and aerospace machinery.
Keynote Forum
Gurdip Singh
Syracuse University, USA
Keynote: Data-driven management of infrastructure systems in smart communities
Time : 10:55-11:25
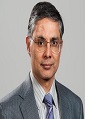
Biography:
Gurdip Singh is the Associate Dean for Research and Graduate Programs at Syracuse University. He was a Program Director at National Science Foundation from 2014 to 2016. From 2009 and 2014, he was the Head of Computer Science Department at Kansas State University. His research interests include real-time embedded systems, sensor networks, network protocols and distributed computing. His research has been funded by NSF, ARO, DARPA and Lockheed Martin. He received his PhD in 1991 for Stony Brook University and BTech from IIT Delhi in 1986.
Abstract:
Development of Smart and Connected Communities will require novel approaches to design reliable and robust infrastructure systems. In addition, to provide resilient services, the interactions and interdependence of infrastructure systems in different domains (e.g., energy, transportation, and public health) must be addressed. This is also resulting in the accumulation of large amounts of data, which can be analyzed, interpreted, and appropriately leveraged. In this presentation, we provide our perspectives on data-driven infrastructure systems in the context of smart and connected communities. We will discuss the need to integrate data from multiple infrastructure systems, and a multidisciplinary approach to address problems in smart communities. We will discuss this in the context of the management of water and road infrastructure systems in a city.
Keynote Forum
Xiaofeng Shao
University of Illinois at Urbana-Champaign, USA
Keynote: Martingale difference divergence and its applications to contemporary statistics
Time : 11:25-11:55
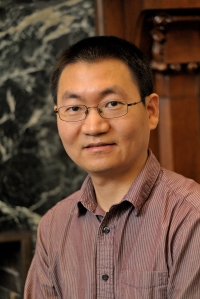
Biography:
Xiaofeng Shao is a professor of Statistics and PhD program director at the Department of Statistics, the University of Illinois at Urbana-Champaign. His main research interests include time series analysis, high dimensional statistics, resampling methods, spatial statistics, and functional data analysis. He is a recipient of The Tjalling C Koopmans Econometric Theory Prize in 2009, Econometric Theory Multa Scripist Award in 2011 and was named as UIUC LAS Centennial Scholar in 2013. He is also an associate editor for the Journal of the American Statistical Association, Journal of Multivariate Analysis and Journal of Time Series Analysis.
Abstract:
Martingale difference divergence is a metric that quantifies the conditional mean dependence of a random vector Y given another random vector X and it can be viewed as an extension of distance covariance, which characterizes the dependence and has recently much attention in the literature. We shall present applications of martingale difference divergence and its variant to several contemporary statistical problems: high dimensional variable screening, dependence testing and dimension reduction for multivariate time series.
- Data Analytics | Big Data Applications | Internet of things | Data Mining Applications in Science, Engineering, Healthcare and Medicine | Cloud computing & E-commerce | Data Mining and Machine Learning | Artificial Intelligence | Biostatistics Application | Statisticsal Methods | Data Mining analysis | Modern Data Analytics | Clinical Biostatistics | Regression Analysis
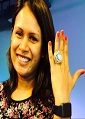
Chair
Subbulakshmi Padmanabhan
StubHub, USA
Session Introduction
Gadde Srinivasa Rao
The University of Dodoma, Tanzania
Title: Better monitoring of diabetic levels using new control charts
Time : 15:05-15:30
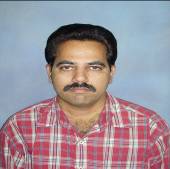
Biography:
Gadde Srinivasa Rao received his MSc in Statistics (1988), MPhil.in Statistics (1994) and PhD in Statistics (2002) from the Acharya Nagarjuna University, Guntur, India. He is presently working as Professor of Statistics at the Department of Statistics, The University of Dodoma, Tanzania. He boasts more than 90 publications in different peer-reviewed journals in national and international well reputed journals including for example, Journal of Applied Statistics, International Journal of Advanced Manufacturer Technology, Communications in Statistics-Theory and methods, Communications in Statistics-Simulation and Computation, Journal of Testing and Evaluation, Arabian Journal for Science and Engineering, International Journal of Quality & Reliability Management, Economic Quality Control and Journal of Statistical Computation and Simulation. He is the reviewer for various reputed international journals. His research interests include statistical inference, statistical process control, applied Statistics, acceptance sampling plans and reliability estimation.
Abstract:
The diabetes monitoring is very important to maintain the diabetic levels in control. The Shewhart control chart has been widely used in the healthcare department for the monitoring of sugar levels. In this paper, we will present a more efficient way to monitor diabetic level in diabetes patients. The structure of control is presented using repetitive sampling. The efficiency of the proposed chart in detecting a shift in diabetic level is compared with the existing chart. It is found that the proposed chart provides a strict way to monitor the diabetic levels in diabetes patients. So, the application of the proposed chart is shown using simulation study and real data collected from diabetes patient. It can be concluded that the use of the proposed chart in health care issue may reduce the risk of heart disease by monitoring diabetic levels in an effective way.
Abdul Basit
State Bank of Pakistan, Pakistan
Title: Estimation of differencing parameter of arima models
Time : 15:30-15:55
Biography:
Abdul Basit is the PhD Research Scholar in the discipline of Statistics in National College of Business Administration and Economics Lahore, Pakistan. He has completed his MS in Social Sciences from SZABIST Karachi, Pakistan in 2014. Currently, he is serving as Deputy Director in Research Cluster of State Bank of Pakistan. He has published 07 research papers in journals and many articles were presented at national and international conferences.
Abstract:
Forecasting of key economic indicators has an important role in the policymaking. Statisticians and economist are still trying to find out the techniques and models which provides a more accurate forecast. There are different time series models are available in the literature like Auto-Regressive (AR) model, Moving Average (MA) model, Auto-Regressive Moving Average (ARMA) model, Auto-Regressive Integrated Moving Average (ARIMA) model, Auto-Regressive Fractionally Integrated Moving Average (ARFIMA) model, and many others. ARIMA and ARFIMA mostly used for the analysis of time series. In this study, we are trying to estimate the differencing parameter’ using the information function and entropy. The comparison of classical time series models and a new time series model is also included in this study. The new estimator of the differencing parameter will give us a more accurate forecast as compared to the classical time series models.
Sumith Gunasekera
The University of Tennessee at Chattanooga, USA
Title: Exact inference for the Youden index to discriminate individuals using twoparameter exponentially-distributed pooled samples
Time : 16:15-16:40
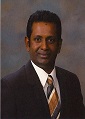
Biography:
Sumith Gunasekera received the (Special Bachelor of Science) B.Sc.(Sp.) degree in Physics in 1995 from the University of Colombo, Colpetty, Colombo 03, District of Colombo, Western province, Democratic Socialist Republic of Sri Lanka (DSRSL) (formerly known as Ceilão (in Portuguese under their rule), Seylon (by Dutch under their rule), and Ceylon (by British under their rule), and the (Doctor of Philosophy) Ph.D. degree in Statistics in 2009 from the University of Nevada at Las Vegas (UNLV), Las Vegas, NV, United States of America (USA). Sumith joined the Department of Mathematics at The University of Tennessee at Chattanooga, Chattanooga TN, the USA in 2009, and has been an Associate Professor of Statistics since 2015. He is the author of many seminal statistical articles and is the recipient of several grants and awards. His research interests include statistical inference, reliability, survival analysis, the design of experiments under classical, Bayesian, and generalized frameworks.
Abstract:
It has become increasingly common in epidemiological studies to pool specimens across subjects as a useful cost-cutting technique to achieve accurate quantification of biomarkers and certain environmental chemicals. The data collected from these pooled samples can then be utilized to estimate the Youden Index (or Youden Statistic) developed by Youden (Youden WJ. Index for rating diagnostic tests. Cancer 1950;3(1):32– 35.), which measures biomarker’s effectiveness and aids in the selection of an optimal threshold value, as a summary measure of the Receiver Operating Characteristic (ROC) curve. The aim of this paper is to make use of generalized approach due to Tsui and Weerahandi ( Tsui K, Weerahandi, S. Generalized p-values in significance testing of hypotheses in the presence of nuisance parameters. J. Amer. Statist. Assoc. 1989;84(406):602–607.) to estimate and testing of the Youden index. This goal is accomplished by the comparison of classical and generalized procedures for the Youden Index with the aid of pooled samples from the shifted-exponentially distributed biomarkers for the low-risk and high-risk patients. These are juxtaposed using confidence intervals, p-values, the power of the test, the size of the test, and coverage probability with a wide-ranging simulation study featuring a selection of various scenarios. In order to demonstrate the advantages of the proposed generalized procedures over its classical counterpart, an illustrative example is discussed using the Duchenne Muscular Dystrophy (DMD) data available at
http://biostat.mc.vanderbilt.edu/wiki/Main/DataSets or http://lib.stat.cmu.edu/datasets/.
- Data Analytics | Big Data Applications | Internet of things | Data Mining Applications in Science, Engineering, Healthcare and Medicine | Cloud computing & E-commerce | Data Mining and Machine Learning | Artificial Intelligence
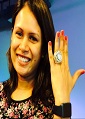
Chair
Subbulakshmi Padmanabhan
StubHub, USA
Session Introduction
Asif Ali
State Bank of Pakistan, Pakistan
Title: Data mining via entropy and time series analysis
Time : 13:50-14:15
Biography:
Asif Ali has completed MS degree in Applied Economics in 2014. He has also sufficient knowledge in the discipline of Statistics with additional degree of MSc (Statistics). Currently he is working in Central Bank of Pakistan in the International Trade section.
Abstract:
Entropy, a mathematical tool used to gather maximum information regarding understudying distribution, systems, databases and surveys. We are introducing entropy as a tool which provides the maximum information about the trading behaviour in different regions. This study will lead us to explore the new avenues of business and investment in Pakistan. China is the biggest player in global trade from the Asian region. To expand the scope of competitiveness, China is continuously investing in the world. The China–Pakistan Economic Corridor (CPEC) is one of the major projects. An extension of China's economic ambition One Belt-One Road initiative (OBOR). In future, China wants to expand her trade with the world using the CPEC to enhance the scope of competitiveness. Pakistan also believes in open trade and continuously trying to enhance trade with the world. To attain maximum advantage of CPEC, to explore the opportunities for the investors and business communities. In this study, we will develop linkages between the trends of our industries, commodities and their future demand in different regions.
Olawale Abolade O
Federal Polytechnic, Nigeria
Title: Effect of competing for risk among breast cancer
Time : 14:15-14:40
Biography:
Olawale Abolade O is a Lecturer in the Department of Statistics, Federal Polytechnic, Ile-Oluji, Ondo State, Nigeria. She holds an MSc Degree in Statistics. Mrs. Olawale is a member of many Professional bodies among which are International Biometry Society (IBS), Nigerian Statistical Association (NSA), Nigerian Mathematical Society (NMS). She has attended so many conferences, seminars and workshops both locally and abroad to update her knowledge in which she has presented some of her works. Presently, she is a PhD Student in the Department of Statistics, University of Ilorin, Nigeria. Her research area is Bio-statistics with a special interest in Survival Analysis.
Abstract:
A retrospective data set was obtained from a government University Teaching Hospital for breast cancer patients classified by the cause of death. The first cause of death was cancer while the other cause arose from any other, all of which were put together and referred to as the competing risk. Appropriate probability distributions were fitted to the time-to-death data of the two groups. The three-parameter Weibull distribution was appropriate to the patients who died of cancer, while exponential probability distribution fitted the breast cancer patients who died of other causes. The implication of this distribution is that survival chance changes between the two competing risks such that overtime other causes of death overshadows death arising from breast cancer.
Tamer Elsayed Ahmed Said
National Research Centre, Egypt
Title: Application of Artificial Intelligence techniques to formulate a mathematical equation for the uniaxial compressive strength of fly ash concrete
Time : 14:40-15:05
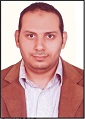
Biography:
Tamer Elsayed has completed his PhD in the year 2014 from the faculty of Engineering Cairo University. His PhD entitled “Development of an artificial neural network to predict the concrete deterioration due to chemical attack”. He is a supervisor for many MSc students. He is a member of many research projects. He has published many papers. One of his published papers entitled “Evaluation of field concrete deterioration under real conditions of seawater attack”, Construction and Building Materials 119 (2016):130–144).
Abstract:
In this study, a multi-gene genetic programming (MGGP) and artificial neural network (ANN) techniques are utilized to create two models for prediction of concrete uniaxial compressive strength. Concrete is a highly complicated heterogeneous material and a precise model of its uniaxial compressive strength is highly nonlinear. Due to the importance of concrete uniaxial compressive strength as the most important characteristic of concrete, converting gathered experimental data from literature to a user-friendly formula is strongly needed for concrete mix design purpose and consequently structural analysis applications. The proposed mathematical expression links the concrete ingredients such as water content, super-plasticizer content, cement content, fly ash content, etc., as inputs and uniaxial compressive strength as output. The results indicated that the created MGGP model and ANN model are precisely able to predict the concrete uniaxial compressive strength in close agreement with the experimental results. Finally, the process of formulation of mathematical equations utilized in this study is a useful guideline in data fitting applications.
- Workshop
Session Introduction
Ergi Sener
IdeaField BV, Holland
Title: Disrupting retail analysis with AI powered advanced analytics solution - Idea Field BV
Time : 15:05-16:00
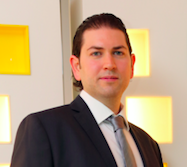
Biography:
Ergi Sener, who is indicated as one of the 20 Turkish people to be followed in the field of technology, received a BS in Microelectronics Engineering in 2005 and double MS in Telecommunications & Management in 2007 from Sabanci University. He is pursuing a PHD degree in Technology Management & Innovation. He began his career as the co-founder and business development director of New Tone Technology Solutions in 2007 with the partnership of Sabancı University's Venture Program. Between 2009 and 2013, he worked as a CRM specialist at Garanti Payment Systems. In 2013, he joined MasterCard as a business development and innovation manager. He was also one of the co-founders and the managing director of Metamorfoz ICT, a new generation Fintech company and Bonbon Tech, the leader IoT focused new generation analytics company. He is currently acting as the Executive Board Member & CDO of a Dutch-based incubation center IdeaFiedl BV. During his career, along with many others he received “Global Telecoms Business Innovation Award" in 2014, "MasterCard Europe President's Award for Innovation" in 2013, "Payment System of the Year Award" by Payment Systems Magazine in 2012, and "Best Mobile Transaction Solution Award" by SIMagine in 2011.
Abstract:
IdeaField is a disruptive technology innovation that understands and analyses in-store customer behavior (wait time, service time, visit frequencies etc.) without being connected to any wi-fi, or open Bluetooth or without having a smart phone application. Data is collected from wi-fi mode-on mobile devices at the locations, where IdeaField sensors have been deployed. With its unique technology, IdeaField aims to perform real-time behavior-based analysis.
In this workshop, a detailed IdeaField demonstration will be shown with covering all use cases such as employee tracking, queue management, real-time heat maps, location based campaigns, convergence analytics, reporting and other consultancy cases. Also, competitive analysis based on other micro-location based technologies (such as cameras, beacons, sensors, etc) will be covered.
- Poster
Session Introduction
Yongxiang Gao
Sun yat-sen University, China
Title: Classification of multi-channel electroencephalogram time series with linear discriminant analysis
Time : 16:15-16:40
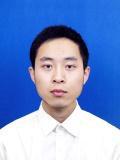
Biography:
Yongxiang Gao has been studying full-time in the graduate program for Master’s Degree on Epidemiology and Health Statistics in the School of Public Health, Sun Yat-sen University from September 2016 to now. The normal study period is three years.
Abstract:
Epilepsy is a chronic neurological disease characterized by epileptic seizures that affect approximately 50 million people worldwide. An electroencephalogram is the most dominant method to detect epileptic seizures, it contains information about brain activity. Therefore, an automatic diagnostic method needed to be proposed to help the doctor make the correct decision, many methods have also been developed during the past years but there is no unanimous opinion. In this work, a strategy has been proposed to differential EEG as normal, epileptic seizures and interictal. Maximal overlap wavelet transform was used to extract wavelet coefficients, five features (variance, Pearson correlation coefficient, Hoeffdings’D measure, Shannon entropy, interquartile range) were calculated from EEG and then input to the linear discriminant classifier for the classification purpose. Data were collected from the Department of Neurology, the Second Affiliated Hospital of Guangzhou Medical University containing 34 healthy people, 30 epileptic seizures patients, and 21 interictal patients. Here only db4 was used. The performance of classifiers was evaluated use leave-one-out cross-validation in terms of accuracy and auc. Results show that the accuracy of healthy and epileptic seizures is 1 and auc is 1. The accuracy of interictal and epileptic seizures is 92.16% and auc is 0.96. The method we proposed can extract information from EEG.