Day 1 :
Keynote Forum
En Bing Lin
Central Michigan University, USA
Keynote: Big data analysis in bioinformatics
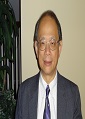
Biography:
En-Bing Lin is a Professor of Mathematics at Central Michigan University, USA. He has been associated with several institutions including Massachusetts Institute of Technology, University of Wisconsin-Milwaukee, University of California, Riverside, University of Toledo, UCLA, and University of Illinois at Chicago. He has received his PhD from Johns Hopkins University. His research interests include Data Analysis, Applied and Computational Mathematics, and Mathematical Physics. He has Supervised a number of graduate and undergraduate students. He serves on the Editorial Boards of several journals. He has organized several special sessions at regional IEEE conferences and many other professional meetings
Abstract:
With the increasing use of advanced technology and the exploding amount of data in bioinformatics, it is imperative to introduce effective and efficient methods to handle Big data using the distributed and parallel computing technologies. Big data analytics can examine large data sets, analyze and correlate genomic and proteomic information. In this presentation, we begin with an overview of Big data and Big data analytics, we then address several challenging and important tasks in bioinformatics such as analyzing coding, noncoding regions and finding similarities for coding and noncoding regions as well as many other issues. We further study mutual information-based gene or feature selection method where features are wavelet-based; the bootstrap techniques employed to obtain an accurate estimate of the mutual information and other new methods to analyze data. Given the multi-scale structure of most biological data, several methods will be presented to achieve improvements in the quality of mathematical or statistical analysis of such data. In a DNA strand, it is essential to find sequences, which can be transcribed to complementary parts of the DNA strand. We will mention several methods to identify protein coding regions. We also use some special variance and entropy to analyze similarities among coding and noncoding regions of several DNA sequences respectively and compare the resulting data. We will address the use of big data analytics in many phases of the bioinformatics analysis pipeline
Keynote Forum
Abdel-Salam Gomaa
Qatar University, Qatar
Keynote: Model robust profile monitoring for the generalized linear mixed model for phase I analysis
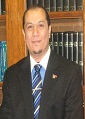
Biography:
Abdel-Salam Gomaa holds BS and MS (2004) degrees in Statistics from Cairo University and MS (2006) and PhD (2009) degrees in Statistics from Virginia Polytechnic Institute and State University (Virginia Tech, USA). Prior to joining Qatar University as an Assistant Professor and a Coordinator of the Statistical Consulting Unit and Coordinator for the Statistics Programs, he taught at Faculty of Economics and Political Science (Cairo University), Virginia Tech, and Oklahoma State University. Also, he worked at JPMorgan Chase Co. as Assistant Vice President in Mortgage Banking and Business Banking Risk Management Sectors. He has published several research papers and delivered numerous talks and workshops. He has awarded couples of the highest prestige awards such as Teaching Excellence from Virginia Tech, Academic Excellence Award, Freud International Award, and Mary G Natrella Scholarship from American Statistical Association (ASA) and American Society for Quality (ASQ), for outstanding graduate study of the theory and application of Quality Control, Quality Assurance, Quality Improvement, and Total Quality Management. He is a Member of the ASQ and ASA
Abstract:
There are so many applications for detecting the changes in the relationship between the response variable and explanatory variable (s) may be the most important consideration rather than detecting the changes in univariate or multivariate quality characteristics. This relationship between the response variable and one or more explanatory variables is called a profile. The act of using various techniques to statistically monitor the process or product profiles is known as profile monitoring. The study introduces two mixed model methods to monitor profiles from the exponential family: a nonparametric (NP) regression method based on the penalized spline regression technique and a semi-parametric (SP) method (Model robust profile monitoring for the generalized linear mixed model (MRGLMM)) which combines the advantages of both the parametric and nonparametric methods. A correctly specified parametric (P) model will have the most power in detecting the profile shift, while a NP method can give improved performance for any type of profile. The MRGLMM method gives results similar to the P method when P model is correctly specified and it gives results similar to the NP method if the proposed P model is badly misspecified. The MRGLMM method gives results that are superior to either the P method or the NP method if the P model provides some useful information regarding profile behavior. Thus, the MRGLMM method is robust to model misspecification. The performances of P, NP and MRGLMM methods are compared through a simulation study using binary data
Keynote Forum
Hong Lin
University of Houston-Downtown, USA
Keynote: Information technologies: opportunities and challenges in personal healthcare systems
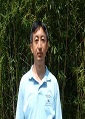
Biography:
Hong Lin was a Postdoctoral Research Associate at Purdue University; an Assistant Research Officer at the National Research Council, Canada, and a Software Engineer at Nokia, Inc. He is currently a Professor with UHD. His research interests include human-centered computing, parallel/distributed computing, grid computing, multi-agent systems, and high level computational models. He is a Co-supervisor of the Grid Computing Lab at UHD
Abstract:
The well-being of a person consists of 2 aspects: the physical body well-being and the mind well-being (the perception or the feeling of well-being). Technology development makes it possible to massively produce cheap sensors for personal use. The data collected, if being properly analyzed, can provide objective and comprehensive personal health information. The information helps us to understand the well-being of the person and then further offers the opportunity to develop a high quality personal healthcare system for the well-being of the person. In this talk, I will report our preliminary findings in applying modern information technology to personal healthcare systems. We construct a brain activity level model by using EEG signals to objectively measure the effectiveness of meditation, detect mental fatigue and boredom, and comprehend human emotions. Also, we have used accelerometer and GPS data to assess sports performance and training enhancement, leg muscle injury prevention and recovery monitoring, and fall prevention for aged people. In addition, the ubiquitous nature of accelerometer and GPS technology make it possible to deliver personal healthcare services for people in physical excise. Then, we exploit the potential of Kinect device in monitoring the movements of aged persons in their houses to prevent falls. Finally, we point out some remaining challenges and possible opportunities in using information technologies to deliver personal health care
- Clinical Biostatistics | Statistical Methods | Bayesian statistics | Biostatistics applications | Big Data Analytics | Structural Bioinformatics | Systems Biology in Bioinformatics | Regression Analysis
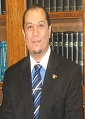
Chair
Abdel-Salam Gomaa
Qatar University, Qatar
Co-Chair
Meenakshi Nadimpalli
Reliable Software Resources Inc., USA
Session Introduction
Pratool Bharti
University of South Florida, USA
Title: HuMAn: complex activity recognition with multi-modal multi-positional body sensing
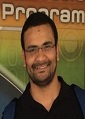
Biography:
Pratool Bharti is pursuing his PhD at University of South Florida, Tampa. He is also a Graduate Student Ambassador for artificial intelligence at Intel corp. He has completed his undergraduate in Computer Science and Engineering from Kalyani Government Engineering College, India in 2010. Before starting his PhD in 2014, he has worked 4 years as Software Developer in machine learning. His current research is in finding pervasive solution for healthcare problems in society. He has published multiple journals and conference papers on activity recognition and smart healthcare
Abstract:
Current state-of-the-art systems in the literature using wearables are not capable of distinguishing many fine-grained and/or complex human activities, which may appear similar but with vital differences in context, such as lying on floor vs. lying on bed vs. lying on sofa. This paper fills this gap by proposing a novel system, called HuMAn, that recognizes and classifies complex at-home activities of humans with wearable sensing. Specifically, HuMAn makes such classifications feasible by leveraging selective multi-modal sensor suites from wearable devices, and enhances the richness of sensed information for activity classification by carefully leveraging placement of the wearable devices across multiple positions on the human body. The HuMAn system consists of the following components: Practical feature set extraction from specifically selected multi-modal sensor suites; a novel two-level structured classification algorithm that improves accuracy by leveraging sensors in multiple body positions; and improved refinement in classification of complex activities with minimal external infrastructure support (e.g., only a few Bluetooth beacons used for location context). The proposed system is evaluated with 10 users in real home environments. Experimental results demonstrate that the HuMAn can detect a total of 21 complex at-home activities with high degree of accuracy. For same-user evaluation strategy, the average activity classification accuracy is as high as 97% over all the 21 activities. For the case of 10-fold cross- validation evaluation strategy, the average classification accuracy is 94%, and for the case of leave-one-out cross-validation strategy, the average classification accuracy is 76%.
Rashid Ahmed
University of North Dakota, USA
Title: Cost analysis of metastatic non-small cell lung cancer (NSCLC) after completion of chemotherapy with the introduction of erlotinib
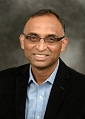
Biography:
Rashid Ahmed has completed his PhD in Bio-Statistics from University of Waterloo; ON; Canada. He has strong background in epidemiology and biostatistics and has experience in the development of statistical methods for the design of community-based interventions and the analysis of longitudinal health data. Currently, he is developing diagnostic measures for joint models for longitudinal and survival data in the presence of non-ignorable missing data. Currently, he is working as an Associate Dean for Research with the College of Nursing and Professional Disciplines at the University of North Dakota. He has published more than 30 papers in reputed journals and has been serving as reviewer of several journals
Abstract:
Erlotinib has been funded for use as a second and third line treatment of advanced NSCLC since 2006 in Manitoba, Canada. Prior research examined, the cost effectiveness of Erlotinib across various countries, yet results may not be generalizable to the healthcare system within Canada. The present paper outlines the cost effectiveness of Erlotinib by examining population-based total costs, service utilization, and clinical outcomes of patients with metastatic NSCLC receiving Erlotinib were explored from the time they completed chemotherapy till the end of follow-up date (31 Dec 2012), death, or relocation. Metastatic NSCLC patients, who were approved for Erlotinib in Manitoba between June 2006 and 31 Dec 2012, were selected. The Manitoba Cancer Registry (MCR) and chart review were used to capture the information on treatment and clinical outcome. Service utilization information and direct cost information were extracted from the electronic health records known as ARIA, the Physicians Claims, the Hospital Discharge database, and the Drug Program Information Network. Survival rate, using the Kaplan-Meier method, was calculated from the date of the Erlotinib approval date till end of follow-up date, Dec 31, 2012, or death. The median survival rate was 40.1 weeks. The average cost per patient was CA$ 30,503 and CA$ 987 per patient-week for patients who received Erlotinib. Ninety percent of the cost was accounted for by hospital stays and drug costs. General demographic patterns per cost suggested that current smokers tended to incur higher costs compared to non-smokers. The results of this study appear to replicate patterns of other studies examining the cost effectiveness such that Erlotinib appears to have a high survival rate paired with lower costs related to side effect management
Owen P L Mtambo
Namibia University of Science and Technology, Namibia
Title: Spatio-temporal quantile interval regression using R-INLA with applications to childhood overweight and obesity in Sub-Saharan Africa
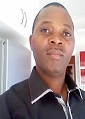
Biography:
Owen P L Mtambo is a Lecturer in Statistics at Namibia University of Science and Technology since March 2014. He was a Lecturer in Statistics at University of Malawi from July 2007 to March 2014. He was Secondary School Teacher in Mathematics and Physics from January 2002 to June 2007. He holds an MSc (Biostatistics) with credit (2012), a BSc (Mathematical Sciences) with distinction (2007), and a DipEdu (Sciences) with distinction (2001); all obtained from University of Malawi. He has more than 8 publications. Currently, he is pursuing his PhD in Statistics at University of South Africa (UNISA) since February 2016.
Abstract:
Childhood malnutrition has serious adverse effects on a child, a family and the development of a country. It leads to more than 30% of deaths in children below five years in sub-Saharan African countries. A malnourished child is more likely to be sick and die. Malnutrition can lead to stunted growth, overweight and obesity, impaired cognitive and behavior development, poor school performance, lower working capacity and lower income. It can slow down economic growth and increase level of poverty. Furthermore, it can prevent a society or a nation from meeting its full potential through loss in productivity, cognitive capacity and increased cost in health care. The indicators of malnutrition range from stunting, wasting and underweight to overweight and obesity. In the past, childhood undernutrition was used to be the most malnutrition burden over the past two decades across the sub-Saharan Africa and is still remaining a burden to date. Doubly surprising, childhood overnutrition is alarmingly becoming the most prevalent parallel to still existing undernutrition burden in sub-Saharan Africa. Overweight and obesity rates are reaching epic proportions in sub-Saharan Africa. The prevalence of childhood overweight and obesity in sub-Saharan Africa was 8.5% in 2010 and is expected to reach 12.7% by 2020. The consequences of overnutrition can be more devastating than those for undernutrition because it leads to chronic failure problems which in turn lead to increased medical expenditure. For this reason, only childhood overweight and obesity were analyzed in this study, in order to assess socio-demographic and socio-economic determinants of childhood overweight and obesity in sub-Saharan Africa. This study also assessed the geographical variation of childhood overweight and obesity in sub-Saharan Africa with more emphasis on both spatial and spatio-temporal effects. All available Demographic and Health Survey (DHS) datasets since 2000 were used and the statistical inference was fully Bayesian using R-INLA package in the selected countries. Almost all studies on spatial quantile modelling of childhood overweight and obesity have emphasized on selecting only one specific response quantile level of interest and report the recommendations based on the only chosen response quantile. Unlike mean response modelling, quantile regression yields model estimates which are stochastic functions of quantile levels such that. This implies that quantile regression modelling using estimates based on only one chosen quantile level might be inefficient and not robust enough. In this study, we used weighted mean estimates based on all quantiles in the quantile interval which corresponded to modelling childhood overweight and obesity. We found out that the significant determinants of childhood overweight and obesity ranged from socio-demographic factors such as type of residence to child and maternal factors such as child age, duration of breastfeeding and maternal BMI. We also observed significant positive structured spatial effects on childhood overweight and obesity mostly in the regions in the center of Namibia.
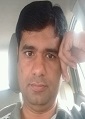
Biography:
Abdul Basit is the PhD Research Scholar in the discipline of Statistics in National College of Business Administration and Economics Lahore, Pakistan. He has completed his MS in Social Sciences from SZABIST Karachi, Pakistan in 2014. Currently, he is serving as Deputy Director in Research Cluster of State Bank of Pakistan. He has published 07 research papers in journals and many articles were presented in national and international conferences
Abstract:
Survival function and hazard rate are very informative and reliable characteristics of any distribution. Entropy is a tool to measure the maximum information of any distribution. In this study, we use the hazard function to develop a new entropy measure. We also introduce the modified forms of Renyi, Tsallis and Havrda and Charvat entropy. The main properties and characteristics and applications associated with these modified entropies are established. We made a comparison between modified entropies by applying them on health indicators Infants Mortality Rate, Crude Death Rate and Life Expectancy of Pakistan. New entropy measures are very useful for censored data. We also introduced a new methodology for the comparison of entropy measures
Mohamed Yusuf Hassan
UAE University, UAE
Title: Integrated quadrbly reduced additive weighted mixture poisson distribution
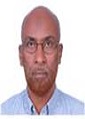
Biography:
Mohamed Yusuf Hassan has completed his PhD from University of California, Riverside. He is the first Author of bivarivarite Mixture Transition Distribution and the Bimodal Skew-Symmetric Normal
Abstract:
We propose a new family of probability distributions derived from the mixtures of weighted Poisson probability distributions. This family of distributions will overcome some of the potential limitations suffered by the existing dominant distributions including lack of modeling over-dispersion, under-dispersion and bimodality. The family is flexible and could be applied to a variety of problems from different disciplines like business, finance, medicine and reliability in engineering. To construct this family, a baseline Poisson distribution and a number of reasonable weights are chosen to get weighted versions of the baseline distribution. These distributions are combined into a mixture format that sums up to a single component probability distribution in a closed form. The resulted density function will be parsimonious and flexible and will be able to capture the nature of many count data patterns. Real count data will be used and goodness-of-fit statistical techniques will be developed to compare its performance with the other existing competing models
- Video Presentation
Session Introduction
Aluko O S
University of KwaZulu-Natal, South Africa
Title: Statistical methodologies for handling ordinal longitudinal responses with monotone dropout patterns using multiple imputation
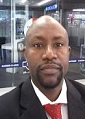
Biography:
Aluko O S is pursuing his PhD at the University of KwaZulu-Natal, South Africa. Three of his papers are in review under reputable journals and the fourth paper is about to be sent to another journal for publication. As a matter of fact, he use and write codes in both R and SAS softwares conveniently
Abstract:
Missing data are common challenge in any longitudinal clinical trials. Multiple imputation is one of the modern methods of handling incomplete data. This approach is applicable to different missing data patterns but sometimes faced with complexity of the type of variables to be imputed and the mechanism underlying the missing values. In this study, we compare the performance of three methods under multiple imputation, namely expectation maximization, fully conditional specification and multivariate normal imputation in the presence of ordinal responses with monotone dropout. We proposed and demonstrated the usefulness of the ordinal negative binomial distribution for ordinal data generation through simulation studies and implementation. However, the real dataset application and simulation studies reveal that the three methods perform equivalently well, thus any of the methods can be recommended for use
- Workshop
Session Introduction
Abdel-Salam Gomaa
Qatar University, Qatar
Title: Parametric regression analysis for Biostatisticians
Biography:
Abdel-Salam Gomaa holds BS and MS (2004) degrees in Statistics from Cairo University and MS (2006) and PhD (2009) degrees in Statistics from Virginia Polytechnic Institute and State University (Virginia Tech, USA). Prior to joining Qatar University as an Assistant Professor and a Coordinator of the Statistical Consulting Unit and Coordinator for the Statistics Programs, he taught at Faculty of Economics and Political Science (Cairo University), Virginia Tech, and Oklahoma State University. Also, he worked at JPMorgan Chase Co. as Assistant Vice President in Mortgage Banking and Business Banking Risk Management Sectors. He has published several research papers and delivered numerous talks and workshops. He has awarded couples of the highest prestige awards such as Teaching Excellence from Virginia Tech, Academic Excellence Award, Freud International Award, and Mary G Natrella Scholarship from American Statistical Association (ASA) and American Society for Quality (ASQ), for outstanding graduate study of the theory and application of Quality Control, Quality Assurance, Quality Improvement, and Total Quality Management. He is a Member of the ASQ and ASA
Abstract:
This short course is designed to provide some of the advanced and common statistical techniques for researches and practitioners. The short course will demonstrate the parametric simple and multiple linear regression models, Logistic Regression, Poisson Regression, ZIP Poisson Regression models and their applications in Biostatistics, Medical, Biology, Business, Mass Communication, Engineering, public health, Epidemiology, pharmaceutical and biomedical fields. These examples and case-studies will be implemented using the SPSS and SAS. Attendees will learn concepts, techniques and some secrets about the followings: How to use the F-test to determine if your predictor variables have a statistically significant relationship with your outcome/response variable? What are the assumptions for linear regression analysis and what you should do to meet these assumptions? Why adjusted R2 is smaller than R2 and what these numbers mean when comparing between several models? For which predictors are the regression coefficients significantly different than zero and how can you select the significant variables? What is the difference between regression and ANOVA and when are they equivalent? When and how to use the Generalized Linear Models with some examples? How to select the best model? What is the general strategy to approach any modeling problem? How to report the results in your research paper/report?
- Poster Presentations
Session Introduction
Estella Chen-Quin
Kennesaw State University, USA
Title: 3D heat maps of cancer mutations in the mitochondrial electron transport system to identify driver domain candidates
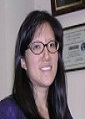
Biography:
Estella Chen-Quin is an Associate Professor in the Department of Molecular and Cellular Biology at Kennesaw State University. She investigates the cancer genetics of mitochondrial lesions during tumor formation. She has completed her PhD at Yale University and Postdoctoral studies at Emory University
Abstract:
A link between tumorigenesis and mutations in the mitochondrial genes of the electron transport system (ETS) has long been posited, due to the high prevalence of mitochondrial mutations in all tumors. However, whether mitochondrial mutations play a causal role in cancer is unknown. Here, we analyze somatic mutations of ETS complex II (Succinate Dehydrogenase; SDH). People with inherited (germline) mutations in SDH have a known cancer predisposition; however, the path from inherited predisposition to cancer-causing lesions is not understood. Mutational lesions in the ETS disrupt the normal flow of electrons, increasing levels of reactive oxygen species (ROS) and creating a mutagenic source. Increased levels of ROS stabilize the mitogenic factor HIF-1ï¡. To identify and visualize which SDH protein domains are selected for somatic missense mutations during tumorigenesis, we created the 3D protein heatmap to identify potential cancer driver domains. A structural analysis via homology modeling allows us to highlight cancer-associated mutations on the ETS protein structure. This may identify areas of the mitochondrial proteins that promote cancer when altered and inform on the cellular mechanisms involved. Mutations are scored for their predicted protein effect using Polyphen2; and potential driver domains are identified by their mean Polyphen2 score. Cancer data is taken from the Cancer Genome Atlas; control data is from the 1000 Genomes Project. We use Pymol and the porcine crystal protein structure to create the 3D protein heatmaps, providing comparative analysis between the control and cancer set
- Clinical Biostatistics | Statistical Methods | Regression Analysis | Systems Biology in Bioinformatics | Biostatistics applications | Biometric security | Big Data Analytics | Modern data analysis
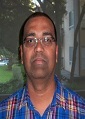
Chair
Yedidi Narasimha Murty
BI Solutions Architect, USA
Co-Chair
Meenakshi Nadimpalli
Reliable Software Resources Inc., USA
Session Introduction
Anam Riaz
National College of Business Administration and Economics, Pakistan
Title: Exponential behavior of health indicators of Pakistan
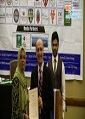
Biography:
Anam Riaz is PhD Research Scholar in the discipline of Statistics in National College of Business Administration and Economics Lahore, Pakistan. Higher Education Commission (HEC) of Pakistan awarded her scholarship for PhD. She has published 02 research papers in journals and many articles were presented in national and international conferences
Abstract:
The study is being to describe the relationship of Basic Medical Staff (BMS) and Life Expectancy (LE) of Pakistan. Another relationship has been developed between Infrastructure (INF) of health sector and Life Expectancy. A bivariate exponential distribution has been developed using the hazard rate and found the correlation coefficients of the BMS and LE and INF and LE. The properties and different characteristics have been derived for new bivariate exponential distribution. Empirical study of new bivariate distribution has been found using the Crude Death Rate, Infant Mortality Rate, Life Expectancy, Medical Staff and Infrastructure of Pakistan
Gbenga J Abiodun
Foundation for Professional Development, South Africa
Title: Examining the effect of climate variability on malaria transmission using a dynamic mosquito-human malaria model
Biography:
Gbenga J Abiodun is a young Scientist whose research interest focuses on biomathematics, epidemiology and mathematical modelling of the impacts of climate (variability and change) on vector-borne and infectious diseases. He has completed his Masters and Doctoral degrees in Mathematics at the University of the Western Cape (UWC) in 2012 and 2016, respectively. He has worked extensively on infectious diseases and published peer-reviewed papers in high-profile international journals
Abstract:
The reasons for malaria resurgence mostly in Africa are yet to be well understood. Although the causes are often linked to regional climate change, it is important to understand the impact of climate variability on the dynamics of the disease. However, this is difficult due to the unavailability of long-term malaria data over the study areas. In this study, we develop a climate-based mosquito-human malaria model to study malaria dynamics in the human population over KwaZulu-Natal, one of the epidemic provinces in South Africa, from 1970-2005. We compare the model output with available observed monthly malaria cases over the province from September 1999 to December 2003. We further use the model outputs to explore the relationship between the climate variables (rainfall and temperature) and malaria incidence over the province using principal component analysis, wavelet power spectrum and wavelet coherence analysis. The model produces a reasonable fit with the observed data and it captures all the spikes in malaria prevalence. Our results highlight the importance of climate factors on malaria transmission and show the seasonality of malaria epidemics over the province. Results from the principal component analyzes further suggest that, there are two principal factors associated with climates variables and the model outputs. One of the factors indicate high loadings on Susceptible, Exposed and Infected human, while the other is more correlated with Susceptible and Recovered humans. However, both factors reveal the inverse correlation between Susceptible-Infected and Susceptible-Recovered humans respectively. Through the spectrum analysis, we notice a strong annual cycle of malaria incidence over the province and ascertain a dominant of one-year periodicity. Consequently, our findings indicate that an average of 0 to 120-day lag is generally noted over the study period, but the 120-day lag is more associated with temperature than rainfall. This is consistence with other results obtained from our analyses that malaria transmission is more tightly coupled with temperature than with rainfall in KwaZulu-Natal province
Biography:
Revathy Duvedi has been in the Pharma/Biotech industry for more than 26 years and have been Icon for more than 10 years. She has been focusing primarily on Oncology therapeutic area for more than 10 years
Abstract:
The purpose of the paper is to share methods and nuances of how to analyze clinical trial data in oncology. The following will be shared: Kaplan-Meier analyses, handling of missing data, competing risks related to overall survival, issues related to proportional hazards model assumption violations, interval censoring and recurrence event analyses. SAS codes and Macros will also be shared. Typically these topics are spread out in various papers. We will provide a consolidated paper documenting all the issues and methods. The intention is for the paper to be used as a roadmap or blueprint on how to approach analyses in oncology trials covering a variety of indications
Mohamad S Hasan
University of Georgia, USA
Title: Covariate rank weighting is a powerful method for prioritizing hypothesis tests in high throughput data
Biography:
Mohamad S Hasan is a PhD candidate in Statistics at the University of Georgia. He has completed his Masters in Statistical Computing from the University of Central Florida. He intends to start his job career as an Assistant Professor in Statistics, Biostatistics, and Bioinformatics
Abstract:
The large scale multiple testing inherent to high throughput biological data necessitates very high statistical stringency and thus true effects in data are difficult to detect unless they have high effect sizes. One solution to this problem is to use independent information to prioritize the most promising features of the data and thus increase the power to detect them. Weighted p-values provide a general framework for doing this in a statistically rigorous fashion. However, calculating weights that incorporate the independent information and optimize statistical power remains a challenging problem despite recent advances in this area. Existing methods tend to perform poorly in the common situation that true positive features are rare and of low effect size. We introduce Covariate Rank Weighting a method for calculating approximate optimal weights conditioned on the ranking of tests by an external covariate. This approach uses the probabilistic relationship between covariate ranking and test effect size to calculate more informative weights that are not diluted by null effects as is common with group-based methods. This relationship can be calculated theoretically for normally distributed covariates or estimated empirically in other cases. We show via simulations and applications to data that this method outperforms existing methods by a large margin in the rare/low effect size scenario and has at least comparable performance in all scenarios
- E-Poster
Session Introduction
G Sh Tsitsiashvili
Far Eastern Federal University, Russia
Title: Factorization of directed graph describing protein network using for research of plants stability to drought and extreme temperatures
Biography:
In this paper, a sequential algorithm of graph nodes classification and their partial order definition are applying to protein network using for a study of the key players required for connecting ABA signaling and ABA-mediated drought and thermo tolerance. Suggested classification procedure allows finding in the network using for research of plants stability to drought and extreme temperatures proteins, the most important clusters for thermo stability and impacts to provide them conditions that are more convenient. It is possible to allocate output proteins DREB2C, ABA receptors PYLs, which are the most important for thermo stability of plants by their biochemical characteristics. In the network, there are only two multi node clusters and only one of them has edges connected with allocated output proteins. Details of this multi node cluster with allocated output proteins are analyzing
Abstract:
G Sh Tsitsiashvili is is professor of the chair of Algebra, Geometry and Analysis at Far Eastern Federal University. 68 years since the birth of Tsitsiashvili Gurami Shalvovich (19 December, 1948), doctor of physical-mathematical sciences, Professor, main scientific researcher of Institute for Applied Mathematics Far Eastern Branch of RAS